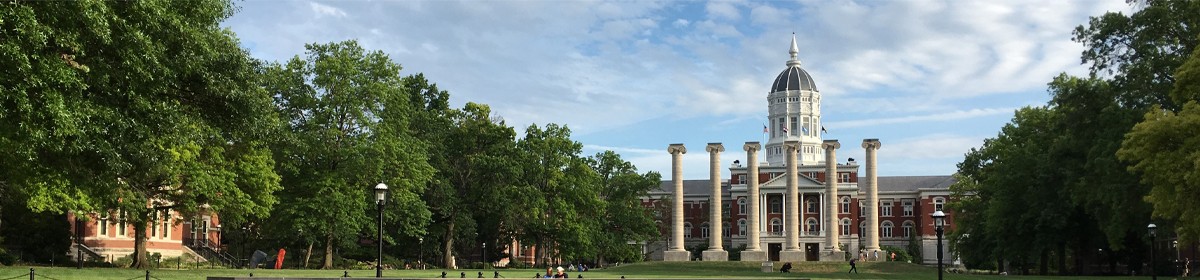
Explosive Hazard Pre-Screener Based on Simulated Data with Perfect Annotation and Imprecisely Labeled Real Data
Proc. SPIE 12116, Chemical, Biological, Radiological, Nuclear, and Explosives (CBRNE) Sensing XXIII, 2022
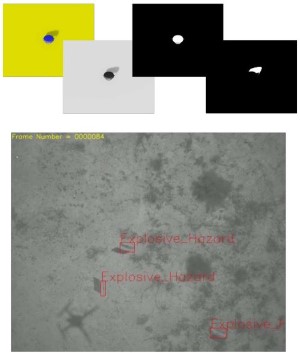
Abstract
Datasets with accurate ground truth from unmanned aerial vehicles (UAV) are cost and time prohibitive. This is a problem as most modern machine learning (ML) algorithms are based on supervised learning and require large and diverse well-annotated datasets. As a result, new creative ideas are needed to drive innovation in robust and trustworthy artificial intelligence (AI) / ML. Herein, we use the Unreal Engine (UE) to generate simulated visual spectrum imagery for explosive hazard detection (EHD) with corresponding pixel-level labels, UAV metadata, and environment metadata. We also have access to a relatively small set of real world EH data with less precise ground truth – axis aligned bounding box labels – and sparse metadata. In this article, we train a lightweight, real-time, pixel-level EHD pre-screener for a low-altitude UAV. Specifically, we focus on training with respect to different combinations of simulated and real data. Encouraging preliminary results are provided relative to real world EH data. Our findings suggest that while simulated data can be used to augment limited volume and variety real world data, it could perhaps be sufficient by itself to train an EHD pre-screener.
Media