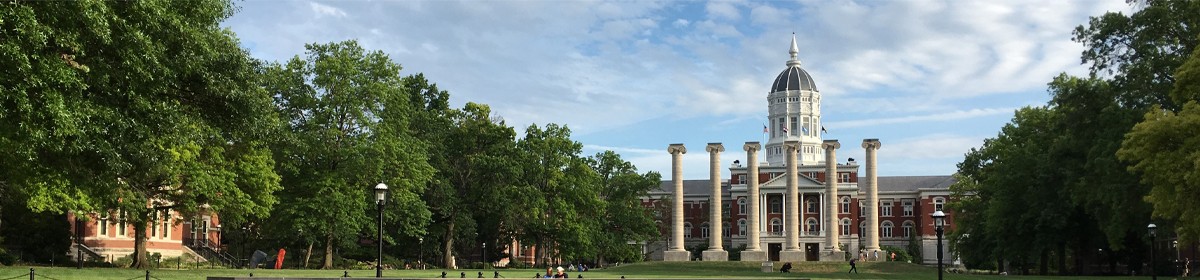
Ignorance Is Bliss: Flawed Assumptions in Simulated Ground Truth
Proc. SPIE 12529, Synthetic Data for Artificial Intelligence and Machine Learning: Tools, Techniques, and Applications, 2023
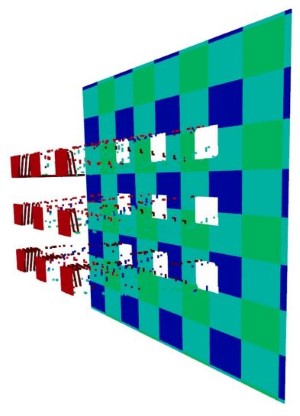
Abstract
Current generation artificial intelligence (AI) is heavily reliant on data and supervised learning (SL). However, dense and accurate truth for SL is often a bottleneck and any imperfections can negatively impact performance and/or result in biases. As a result, several corrective lines of research are being explored, including simulation (SIM). In this article, we discuss fundamental limitations in obtaining truth, both in the physical universe and SIM, and different truth uncertainty modeling strategies are explored. A case study from data-driven monocular vision is provided. These experiments demonstrate performance variability with respect to different truth uncertainty strategies in training and evaluating AI algorithms.
Media